Who knows best where to find what online? Future trends in search
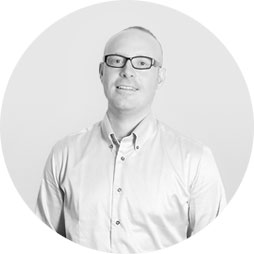
Categories
The internet is often referred to as a massive archive of “human knowledge”. It’s become universally possible to access information on virtually anything – or so it seems. For the average user, finding information can still be a weary process, unless you know how to refine your search queries or have the patience to click through endless links provided by search engines. This can be a tiresome experience, especially if you want quick answers. For a search engine, guessing what a person’s actual intentions are from a few key words still seems quite failure-prone. Sometimes more context is needed to be able to correctly interpret the meaning behind a message, an issue we are all familiar with from everyday language use (see blogpost on NLP).
For these reasons, search engines are trying to help their users find useful answers to information queries by providing tools that do the searching for them. Google for example, the most popular and powerful search engine, introduced its Knowledge Graph, a system of facts about people, places, things, and events, in 2012. The Knowledge Graph quickly pulls information it deems relevant to a search query from different sources and features this information in a panel alongside traditional search results. For example, in response to a search for a particular restaurant, users can find address, menu, opening hours, photos, driving directions as well as links to table booking, reviews and other related info in “featured snippets”. The probability that the desired information is among these snippets is quite high. Recently, even statistics were added such as times of the day during which a venue is most frequented. However, the Knowledge Graph is yet only a resource for very common search queries (according to SEL it is shown in 25% of all search queries).
Nevertheless, when the Knowledge Graph was presented in 2012, it was considered a significant leap in the evolution of search, in particular one that acknowledged that the average internet user requires “answers, not links”. Search engines have undoubtedly become more efficient in predicting user intent, also because of the ability to utilize context information such as a user’s location, search history, and any other information users are willing to share with companies like Google.
In 2016, the number of virtual assistants available to help with queries has exploded. Since the introduction of Apple’s Siri in 2011, almost every major competitor now has a voice-activated personal assistant on the market based on AI technology such as Microsoft’s Cortana or Amazon’s Echo. These products, also referred to as chatbots, acknowledge that the way we interact with the internet has fundamentally changed and has also become increasingly mobile. And advances in natural language processing or voice recognition allow interaction that is like real life. In other words, to “get stuff done” or pull up a quick fact, people are beginning to lead conversations with their smartphones and other devices such as Amazon’s Alexa.
Interacting with bots is basically the same thing as interacting with a search functionality. The difference is that bots engage users in a “conversation”, thereby establishing the additional information needed to bring up a relevant result. In other words, the conversational framework helps the bot narrow down options and search different databases until it comes up with the answer the user is looking for.
Bots can help people in a variety of situations such as checking the weather, booking a table or ordering groceries. A promising area of application is customer service where bots promise to decrease waiting time on-hold by gathering information in advance or even solving issues before a customer is handed over to a human assistant on the phone, thus saving a lot of costs. One of the brands that pioneered the use of Facebook’s Messenger Bot Platform (released in April 2016) for its shopping app is m-commerce clothing retailer Spring. Its “personal shopping assistant” can refine product choices by asking questions about product category and price range until a “personalized” recommendation is displayed including a link to a shopping page (see demo).
In the meantime, however, Spring and many other chatbot pioneering brands have scaled down their bot initiatives or even dropped them completely. Bots still often get things wrong. In fact, according to a recent article on Digiday, Facebook messenger bots have a failure rate of over 70%, leading Facebook to announce plans to “refocus” its AI initiatives. Furthermore, bots cannot show the same amount of options as websites can. So apparently, the artificial intelligence needed to power conversation-based searches has yet to reach a state that generates real business value. Are conversation-based bots thus no more than just another hype? Not necessarily, as lots of analysts agree. As with many applications that are powered by artificial intelligence, success may be further down the line when the bot learns enough through interaction to be able to handle more complex searches.
Recently, a brand-unrelated purpose for bots came to light in a research paper Google published in 2014. The company’s Knowledge Vault, a successor database to the Knowledge Graph, uses bots (or algorithms) to comb through the internet for information it will use “to further scale up knowledge base construction”. These “fact-checking bots” will build the database automatically rather than with editorial help from the crowd, thus promising to be larger than any man-made knowledge database before. Possible uses of the vault are numerous. One is to allow “robots and smartphones to understand what people ask them” or to improve AI performance issues as outlined above. Similar databases are reportedly being built by Facebook, Amazon and Microsoft. These represent “world knowledge” that would be beyond what any human could store and process. Therefore, the question may no longer be who knows how to find what online as the title of this article asks but which algorithm.